
Saturday, August 1, 2009
Wednesday, July 29, 2009
Remote Sensing Module 5:


I sometimes have a hard time differentiating one land type from another. In this case grass/agriculture land covers gave me a lot of trouble. In the Unsupervised map I took that cluster of fairly regular shapes in the north west portion to be an area of High Urban Density. I didn't even include a land cover category for agriculture because I didn't think any sizable farm land existed on the image. Part of my issue was not paying strict attention to the area I was viewing and making assumptions about the image based on past experience. I thought the main body of water running through the center of the map was Escambia Bay, hence I assumed that area in the North West portion to be Pensacola. Nope. That central water body in the image is Perdido Bay making that North West portion of the image a sparsely developed section of Alabama. Upon further review, I took those North West sections to be tracts of farm land. I added the Agriculture land cover category for the Supervised image. I still ended up with a confusing mish mash of land cover types in portions of both maps and agreement between land type categories between each map is sketchy.
Wednesday, July 15, 2009
Remote Sensing Module 4:

Usefulness/Pitfalls of Image Rectification: Once an image has been rectified with a reference, locating points of interest become much easier. In the Pensacola image (above at left) it could be difficult to identify specific buildings, a school for instance. But, after rectifying, the coordinates of a particular school could be taken from the reference map (above at right) and the school could then be pinpointed on the image. A particular issue I had with rectification arose after I placed the first check point. The next one would vanish. I assumed my control points were bad, so I started over with a new set. I ran into the same issue: vanishing check points. I tried them in several places and noticed a distinct pause before rejecting the potential check point. I tried several more until the next one found a permenant home. It required quite a bit of trial and error with check point placement until I had the requisite five sited. My final check point error was 2.27.
Thursday, July 9, 2009
Remote Sensing Module 3:

Roads: The roads are bright because the asphalt paved roads absorb more heat and remain warmer overnight. In addition, the streets have already begun to absorb heat from the early morning (6:45 am) sun.
Natural and man-made vegetation: Overnight cooling of vegetation combined with its higher thermal inertia results in the vegetation being cooler, i.e., darker, in the early morning as it requires a longer period of sun exposure to heat.
Sidewalks and patios: The lower thermal inertia of the concrete allows it to more rapidly heat in the morning light. If the sidewalks and patios are made of certain materials, overnight heat retention may play a role in its brighter appearence, much like the asphalt roads.
Storage sheds in back yards: The sheds appear uniformly dark (cooler), much like the houses. The material from which the sheds were built, wood for instance, would have a higher thermal inertia requiring longer sun exposure for warming. Possibly the materials stored in the sheds may contribute to their cooler thermal appearence. A quantity of stored water or building material within a shed could impact the image. The constant shade provided by the shed would lower the temperature variations the material within experienced thereby increasing solar heating times.
Automobiles: The cars that have been sitting all night appear cooler as the early morning sun has not begun heating them yet. Though, cars that are idling or have recently been operated show hot spots from the heat of the internal combustion engines.
Bright spots on many of the roof tops: Ground Temperature is in the lower fifties (Fahrenheit), so the hot spots are either furnace vents from heating units or chimnies above active fireplaces.
Thursday, July 2, 2009
Remote Sensing Module 2:


The bright blue areas in the multi-spectral image are very hard to recognize in the panchromatic image. When viewed as a color infrared composite the areas stand out in bright red suggesting it must be some type of vegetation. Given this and the fact that the blue colored areas are located in the water, I would guess that they represent a algae bloom, a very dense concentration of seaweed, or some other type of sea plant.
Friday, June 26, 2009
Remote Sensing Module 1:

Tuesday, April 28, 2009
Module 11: Google Earth

Average wind speed at Station MKGM4 at Muskegon, MI (to the northeast of site) is 11 knots (12.7 mph)with gusts of 15 knots (17.3 mph). It's beyond the BERR recommended 200 to 300 meter distance fromdwellings to minimize noise pollution. It is also beyond the BERR recommended 400 to 800 meter distance to avoid adverse reactions to Shadow Flicker. Major shipping routes out of Chicago and Milwaukee are to the southwestand west, respectively. The site is a good compromise with regards to major bird migration corridors,and should be minimally impactful to most species. Its proximity to land should help minimize buildingand transportation costs.
Saturday, April 25, 2009
Before and After
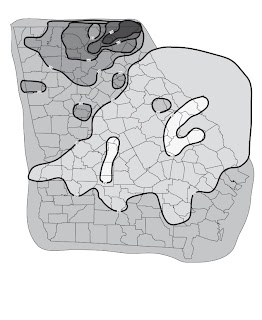
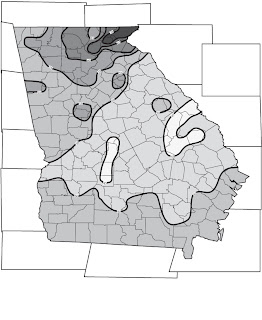
It becomes an exercise in setting up Layers appropriately. It's a bit slow (and tedious). I'm sure there's a more efficient method to do it, but I have not puzzled it out. Good luck!
Friday, April 24, 2009
Sunday, April 12, 2009
Module 9: Flow Maps

Friday, April 10, 2009
Module 8: Contiguous Cartograms

I'm not at all sure about the legend. I wanted to convey that the countries with the greatest increase in size indicated a greater GDP. I used a divergent color scheme to further highlight which countries had higher GDP's. The size increase from the cartogram application made it easy to see which countries had higher GDP's, if I was familiar with the geography. But, a country like South Africa, whose actual shape I'm not terribly familiar with was difficult to determine at a glance.
Failed to include the number of iterations I used. It was 8.
Module 8: Non-Contiguous Cartograms

The biggest problem I had with this map was the projection. If I applied the Robinson World projection, or any other world projections, prior to running the cartogram application, the cartogram output would be at such a large scale it was imperceptible on the small scale world map. I applied the GCS 84, ran the cartogram, then added the Robinson projection. The GCS 84 gives Alaska a stretched out look. The Robinson projection looks more like I expect the world to look. I was guessing on this legend as well. I used the 5 color divergent scheme here, too. So, I added 5 circles. They are only representative of circles that would fall in each division. I prefer the contiguous map. It makes more sense to me visually. In particular, the non-contiguous map is very confusing to look at without the further differentiation a color scheme provides. I made the circles slightly translucent to reveal the base map beneath. It's a bit gaudy looking.
Wednesday, April 1, 2009
Module 7: Dot Distribution Map

I ended up using a dot value of 3, 1 dot is 3 houses per square mile. I used a dot size of 0.6 points, but later added a stroke of 0.2 points. I choose not to display county names, rivers, lakes, or wetlands so the dots would stand out. I found the map very busy looking with all layers displayed. Plus, I had so much fun making 2289 blue dots I just had to show them off :)
Tuesday, March 24, 2009
Friday, March 20, 2009
Choropleth Map Part 2

After coming up with an average population growth for each division, I followed the equal interval formula in the text and came up with a class interval of 4.74%, a high value of 29.6%, and a low value of 5.9%. Equal Interval was probably not the best classification method for this data. The 4th class break, 20.13 - 24.86, is not used. The growth in the mountain division is skewed by Nevada's 66% growth, which is more than twice that of any other mountain division member and, eight times a much as the division slowest growing state, Wyoming at 8.86%. The fact that the entire Northeast and Midwest fell into the first break created a uniformity that's visually unappealing. The grayscale was set manually by percentage in Illustrator. I scaled the divisions down to 75% and moved each around. Seperating each division looked so good on a classmate's blog I had to steal it.
Thursday, March 19, 2009
Choropleth Map Part 1

Friday, March 6, 2009
Tuesday, March 3, 2009
Monday, January 19, 2009
State Preferences
Good map / Bad map
I chose the map of Westeros (#2) from Martin's A Song of Ice and Fire series as an example of a good map and the map of The Six Duchies (#1) from Hobb's Farseer Trilogy as an example of a bad map. Clicking either map will open a seperate window with a better view. Map #1 shows very little detail. Map #2 does a better job of conveying the location of forests, hills, and mountains. The rivers in map #2 and the coastal regions appear in more detail. Map #2 also includes major north/south and east/west roads, while map #1 does not show any travel routes. The second map also includes the names and locations of all cities, towns and landmarks. While reading the books from the Song of Ice and Fire series, I often referenced the map to see where cities lay in relation to other locations visited in the story. Aside from the location of a handful of major towns, the map from The Farseer Trilogy simply didn't offer enough detail to be useful. Perhaps not coincidentally, I enjoyed the Martin series much more than the Hobb series.
Map #1 from: http://crooty.deviantart.com/art/Robin-Hobb-Map-10431866
Map #2 from: http://www.coldones.eu/images/maps/westeros_complete.jpg